Tag: Platform
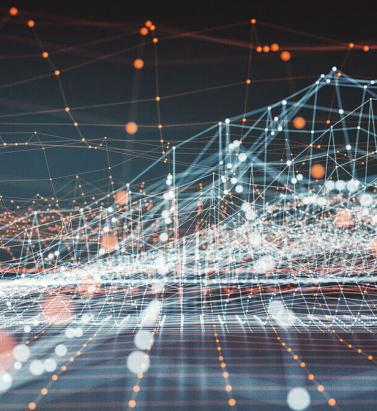
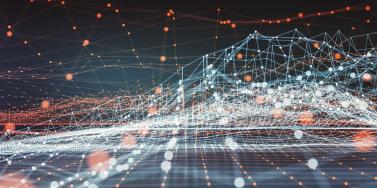
A Solution Shared
May 05, 2020The Risk Data Open Standard is now available, and active industry collaboration is essential for achieving wide-scale interoperability objectives On January 31, the first version of the Risk Data Open Standard™ (RDOS) was made available to the risk community and the public on the GitHub platform. The RDOS is an “open” standard because it is available with no fees or royalties and anyone can review, download, contribute to or leverage the RDOS for their own project. With the potential to transform the way risk data is expressed and exchanged across the (re)insurance industry and beyond, the RDOS represents a new data model (i.e., a data specification or schema) specifically designed for holding all types of risk data, from exposure through model settings to results analyses. The industry has longed recognized that a dramatic improvement in risk data container design is required to support current and future industry operations. The industry currently relies on data models for risk data exchange and storage that were originally designed to support property cat models over 20 years ago. These formats are incomplete. They do not capture critical information about contracts, business structures or model settings. This means that an analyst receiving data in these old formats has detective work to do – filling in the missing pieces of the risk puzzle. Because formats lack a complete picture linking exposures to results, highly skilled, well-paid people are wasting a huge amount of time, and efforts to automate are difficult, if not impossible, to achieve. Existing formats are also very property-centric. As models for new insurance lines have emerged over the years, such as energy, agriculture and cyber, the risk data for these lines of business have either been forced suboptimally into the property cat data model, or entirely new formats have been created to support single lines of business. The industry is faced with two poor choices: accept substandard data or deal with many data formats – potentially one for each line of business – possibly multiplied by the number of companies who offer models for a particular line of business. The industry is painfully aware of the problems we are trying to solve. The RDOS aims to provide a complete, flexible and interoperable data format ‘currency’ for exchange that will eliminate the time-consuming and costly data processes that are currently required Paul Reed RMS “The industry is painfully aware of the problems we are trying to solve. The RDOS aims to provide a complete, flexible and interoperable data format ‘currency’ for exchange that will eliminate the time-consuming and costly data processes that are currently required,” explains Paul Reed, technical program manager for the RDOS at RMS. He adds, “Of course, adoption of a new standard can’t happen overnight, but because it is backward-compatible with the RMS EDM and RDM users have optionality through the transition period.” Taking on the Challenge The RDOS has great promise. An open standard specifically designed to represent and exchange risk data, it accommodates all categories of risk information across five critical information sets – exposure, contracts (coverage), business structures, model settings and results analyses. But can it really overcome the many intrinsic data hurdles currently constraining the industry? According to Ryan Ogaard, senior vice president of model product management at RMS, its ability to do just that lies in the RDOS’s conceptual entity model. “The design is simple, yet complete, consisting of these five linked categories of information that provide an unambiguous, auditable view of risk analysis,” he explains. “Each data category is segregated – creating flexibility by isolating changes to any given part of the RDOS – but also linked in a single container to enable clear navigation through and understanding of any risk analysis, from the exposure and contracts through to the results.” By adding critical information about the business structure and models used, the standard creates a complete data picture – a fully traceable description of any analysis. This unique capability is a result of the superior technical data model design that the RDOS brings to the data struggle, believes Reed. “The RDOS delivers multiple technical advantages,” he says. “Firstly, it stores results data along with contracts, business structure and settings data, which combine to enable a clear and comprehensive understanding of analyses. Secondly, the contract definition language (CDL) and structure definition language (SDL) provide a powerful tool for unambiguously determining contract payouts from a set of claims. In addition, the data model design supports advanced database technology and can be implanted in several popular DB formats including object-relational and SQL. Flexibility has been designed into virtually every facet of the RDOS, with design for extensibility built into each of the five information entities.” “New information sets can be introduced to the RDOS without impacting existing information,” Ogaard says. “This overcomes the challenges of model rigidity and provides the flexibility to capture multivendor modeling data, as well as the user’s own view of risk. This makes the standard future-proof and usable by a broad cross section of the (re)insurance industry and other industries.” Opening Up the Standard To achieve the ambitious objective of risk data interoperability, it was critical that the RDOS was founded on an open-source platform. Establishing the RDOS on the GitHub platform was a game-changing decision, according to Cihan Biyikoglu, executive vice president of product at RMS. You have to recognize the immense scale of the data challenge that exists within the risk analysis field. To address it effectively will require a great deal of collaboration across a broad range of stakeholders. Having the RDOS as an open standard enables that scale of collaboration to occur. “I’ve worked on a number of open-source projects,” he says, “and in my opinion an open-source standard is the most effective way of energizing an active community of contributors around a particular project. “You have to recognize the immense scale of the data challenge that exists within the risk analysis field. To address it effectively will require a great deal of collaboration across a broad range of stakeholders. Having the RDOS as an open standard enables that scale of collaboration to occur.” Concerns have been raised about whether, given its open-source status and the ambition to become a truly industrywide standard, RMS should continue to play a leading role in the ongoing development of the RDOS now that it is open to all. Biyikoglu believes it should. “Many open-source projects start with a good initial offering but are not maintained over time and quickly become irrelevant. If you look at the successful projects, a common theme is that they emanate from an industry participant suffering greatly from the particular issue. In the early phase, they contribute the majority of the improvements, but as the project evolves and the active community expands, the responsibility for moving it forward is shared by all. And that is exactly what we expect to see with the RDOS.” For Paul Reed, the open-source model provides a fair and open environment in which all parties can freely contribute. “By adopting proven open-source best practices and supported by the industry-driven RDOS Steering Committee, we are creating a level playing field in which all participants have an equal opportunity to contribute.” Assessing The Potential Following the initial release of the RDOS, much of the activity on the GitHub platform has involved downloading and reviewing the RDOS data model and tools, as users look to understand what it can offer and how it will function. However, as the open RDOS community builds and contributions are received, combined with guidance from industry experts on the steering committee, Ogaard is confident it will quickly start generating clear value on multiple fronts. “The flexibility, adaptability and completeness of the RDOS structure create the potential to add tremendous industry value,” he believes, “by addressing the shortcomings of current data models in many areas. There is obvious value in standardized data for lines of business beyond property and in facilitating efficiency and automation. The RDOS could also help solve model interoperability problems. It’s really up to the industry to set the priorities for which problem to tackle first. The flexibility, adaptability and completeness of the RDOS structure create the potential to add tremendous industry value “Existing data formats were designed to handle property data,” Ogaard continues, “and do not accommodate new categories of exposure information. The RDOS Risk Item entity describes an exposure and enables new Risk Items to be created to represent any line of business or type of risk, without impacting any existing Risk Item. That means a user could add marine as a new type of Risk Item, with attributes specific to marine, and define contracts that cover marine exposure or its own loss type, without interfering with any existing Risk Item.” The RDOS is only in its infancy, and how it evolves – and how quickly it evolves – lies firmly in the hands of the industry. RMS has laid out the new standard in the GitHub open-source environment and, while it remains committed to the open standard’s ongoing development, the direction that the RDOS takes is firmly in the hands of the (re)insurance community. Access the Risk Data Open Standard here
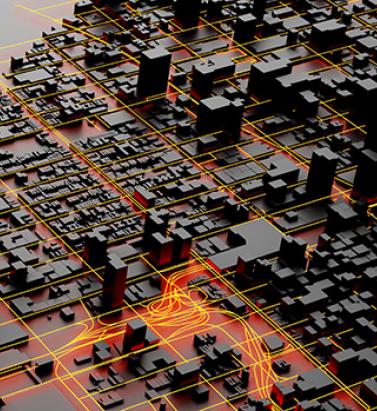
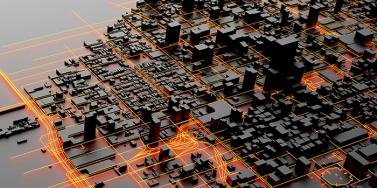
Insurance: The next 10 years
September 06, 2019Mohsen Rahnama, Cihan Biyikoglu and Moe Khosravy of RMS look to 2029, consider the changes the (re)insurance industry will have undergone and explain why all roads lead to a platform Over the last 30 years, catastrophe models have become an integral part of the insurance industry for portfolio risk management. During this time, the RMS model suite has evolved and expanded from the initial IRAS model — which covered California earthquake — to a comprehensive and diverse set of models covering over 100 peril-country combinations all over the world. RMS Risk Intelligence™, an open and flexible platform, was recently launched, and it was built to enable better risk management and support profitable risk selection. Since the earliest versions of catastrophe models, significant advances have been made in both technology and computing power. These advances allow for a more comprehensive application of new science in risk modeling and make it possible for modelers to address key sources of model and loss uncertainty in a more systematic way. These and other significant changes over the last decade are shaping the future of insurance. By 2029, the industry will be fully digitized, presenting even more opportunity for disruption in an era of technological advances. In what is likely to remain a highly competitive environment, market participants will need to differentiate based on the power of computing speed and the ability to mine and extract value from data to inform quick, risk-based decisions. Laying the Foundations So how did we get here? Over the past few decades we have witnessed several major natural catastrophes including Hurricanes Andrew, Katrina and Sandy; the Northridge, Kobe, Maule, Tōhoku and Christchurch Earthquakes; and costly hurricanes and California wildfires in 2017 and 2018. Further, human-made catastrophes have included the terrorist attacks of 9/11 and major cyberattacks, such as WannaCry and NotPetya. Each of these events has changed the landscape of risk assessment, underwriting and portfolio management. Combining the lessons learned from past events, including billions of dollars of loss data, with new technology has enhanced the risk modeling methodology, resulting in more robust models and a more effective way to quantify risk across diverse regions and perils. The sophistication of catastrophe models has increased as technology has enabled a better understanding of root causes and behavior of events, and it has improved analysis of their impact. Technology has also equipped the industry with more sophisticated tools to harness larger datasets and run more computationally intensive analytics. These new models are designed to translate finer-grained data into deeper and more detailed insights. Consequently, we are creating better models while also ensuring model users can make better use of model results through more sophisticated tools and applications. A Collaborative Approach In the last decade, the pace at which technology has advanced is compelling. Emerging technology has caused the insurance industry to question if it is responding quickly and effectively to take advantage of new opportunities. In today’s digital world, many segments of the industry are leveraging the power and capacity enabled by Cloud-computing environments to conduct intensive data analysis using robust analytics. Technology has also equipped the industry with more sophisticated tools to harness larger datasets Such an approach empowers the industry by allowing information to be accessed quickly, whenever it is needed, to make effective, fully informed decisions. The development of a standardized, open platform creates smooth workflows and allows for rapid advancement, information sharing and collaboration in growing common applications. The future of communication between various parties across the insurance value chain — insurers, brokers, reinsurers, supervisors and capital markets — will be vastly different from what it is today. By 2029, we anticipate the transfer of data, use of analytics and other collaborations will be taking place across a common platform. The benefits will include increased efficiency, more accurate data collection and improvements in underwriting workflow. A collaborative platform will also enable more robust and informed risk assessments, portfolio rollout processes and risk transfers. Further, as data is exchanged it will be enriched and augmented using new machine learning and AI techniques. An Elastic Platform We continue to see technology evolve at a very rapid pace. Infrastructure continues to improve as the cost of storage declines and computational speed increases. Across the board, the incremental cost of computing technology has come down. Software tools have evolved accordingly, with modern big data systems now capable of handling hundreds if not thousands of terabytes of data. Improved programming frameworks allow for more seamless parallel programming. User-interface components reveal data in ways that were not possible in the past. Furthermore, this collection of phenomenal advances is now available in the Cloud, with the added benefit that it is continuously self-improving to support growing commercial demands. In addition to helping avoid built-in obsolescence, the Cloud offers “elasticity.” Elasticity means accessing many machines when you need them and fewer when you don’t. It means storage that can dynamically grow and shrink, and computing capacity that can follow the ebb and flow of demand. In our world of insurance and data analytics, the macro cycles of renewal seasons and micromodeling demand bursts can both be accommodated through the elastic nature of the Cloud. In an elastic world, the actual cost of supercomputing goes down, and we can confidently guarantee fast response times. Empowering Underwriters A decade from now, the industry will look very different, not least due to changes within the workforce and the risk landscape. First-movers and fast-followers will be in a position of competitive advantage come 2029 in an industry where large incumbents are already partnering with more agile “insurtech” startups. The role of the intermediary will continue to evolve, and at every stage of risk transfer — from insured to primary insurer, reinsurer and into the capital markets — data sharing and standardization will become key success factors. Over the next 10 years, as data becomes more standardized and more widely shared, the concept of blockchain, or distributed ledger technology, will move closer to becoming a reality. This standardization, collaboration and use of advanced analytics are essential to the future of the industry. Machine learning and AI, highly sophisticated models and enhanced computational power will enable underwriters to improve their risk selection and make quick, highly informed decisions. And this ability will enhance the role of the insurance industry in society, in a changing and altogether riskier world. The tremendous protection gap can only be tackled when there is more detailed insight and differentiation around each individual risk. When there is greater insight into the underlying risk, there is less need for conservatism, risks become more accurately and competitively priced, and (re)insurers are able to innovate to provide products and solutions for new and emerging exposures. Over the coming decade, models will require advanced computing technology to fully harness the power of big data. Underwater robots are now probing previously unmapped ocean waters to detect changes in temperatures, currents, sea level and coastal flooding. Drones are surveying our built-up environment in fine detail. Artificial intelligence and machine learning algorithms are searching for patterns of climate change in these new datasets, and climate models are reconstructing the past and predicting the future at a resolution never before possible. These emerging technologies and datasets will help meet our industry’s insatiable demand for more robust risk assessment at the level of an individual asset. This explosion of data will fundamentally change the way we think about model execution and development, as well as the end-to-end software infrastructure. Platforms will need to be dynamic and forward-looking verses static and historic in the way they acquire, train, and execute on data. The industry has already transformed considerably over the past five years, despite traditionally being considered a laggard in terms of its technology adoption. The foundation is firmly in place for a further shift over the next decade where all roads are leading to a common, collaborative industry platform, where participants are willing to share data and insights and, as they do so, open up new markets and opportunities. RMS Risk Intelligence The analytical and computational power of the Risk Intelligence (RI) platform enables the RMS model development team to bring the latest science and research to the RMS catastrophe peril model suite and build the next generation of high-definition models. The functionality and high performance of RI allows the RMS team to assess elements of model and loss uncertainty in a more robust way than before. The framework of RI is flexible, modular and scalable, allowing the rapid integration of future knowledge with a swifter implementation and update cycle. The open modeling platform allows model users to extract more value from their claims experience to develop vulnerability functions that represent a view of risk specific to their data or to use custom-built alternatives. This enables users to perform a wide range of sensitivity tests and take ownership of their view of risk. Mohsen Rahnama is chief risk modeling officer and executive vice president, models and data, Cihan Biyikoglu is executive vice president, product and Moe Khosravy is executive vice president, software and platform at RMS
