This is a taster of an article published by RMS in the second edition of EXPOSURE magazine. Click here and download your full copy now.
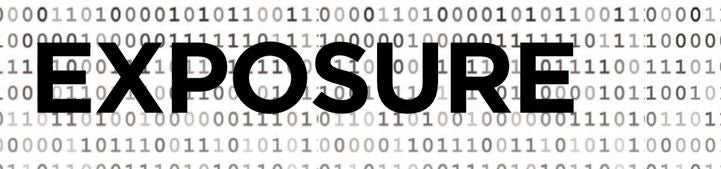
In EXPOSURE magazine, we delved into the algorithmic depths of machine learning to better understand the data potential that it offers the insurance industry. In the article, Peter Hahn, head of predictive analytics at Zurich North America illustrated how pattern recognition sits at the core of current machine learning. How do machines learn? Peter compares it to how a child is taught to differentiate between similar animals; a machine would “learn” by viewing numerous different pictures of the animals, which are clearly tagged, again and again.
Hahn comments “Over time, the machine intuitively forms a pattern recognition that allows them to tell a tiger from, say, a leopard. You can’t predefine a set of rules to categorize every animal, but through pattern recognition you learn what the differences are.”
Hahn adds that pattern recognition is already a part of how underwriters assess a risk. “A decision-making process will obviously involve traditional, codified analytical processes, but it will also include sophisticated pattern recognition based on their experiences of similar companies operating in similar fields with similar constraints. They essentially know what this type of risk ‘looks like’ intuitively.”
The Potential of Machine Learning
EXPOSURE magazine asked Christos Mitas, vice president of model development at RMS, on how he sees machine learning being used. Mitas opened the discussion saying “We are now operating in a world where that data is expanding exponentially, and machine learning is one tool that will help us to harness that.”
Here are three areas where Mitas believes machine learning will make an impact:
Cyber Risk Modeling: Mitas adds “Where machine learning can play an important role here is in helping us tackle the complexity of this risk. Being able to collect and digest more effectively the immense volumes of data which have been harvested from numerous online sources and datasets will yield a significant advantage.”
Image Processing: “With developments in machine learning, for example, we might be able to introduce new data sources into our processing capabilities and make it a faster and more automated data management process to access images in the aftermath of a disaster. Further, we might be able to apply machine learning algorithms to analyze building damage post event to support speedier loss assessment processes.”
Natural Language Processing: “Advances here could also help tremendously in claims processing and exposure management,” Mitas adds, “where you have to consume reams of reports, images and facts rather than structured data. That is where algorithms can really deliver a different scale of potential solutions.”
For the full article and more insight for the insurance industry, click here and download your full copy of EXPOSURE magazine now.